AI System May Speed Accurate Diagnosis of EBA
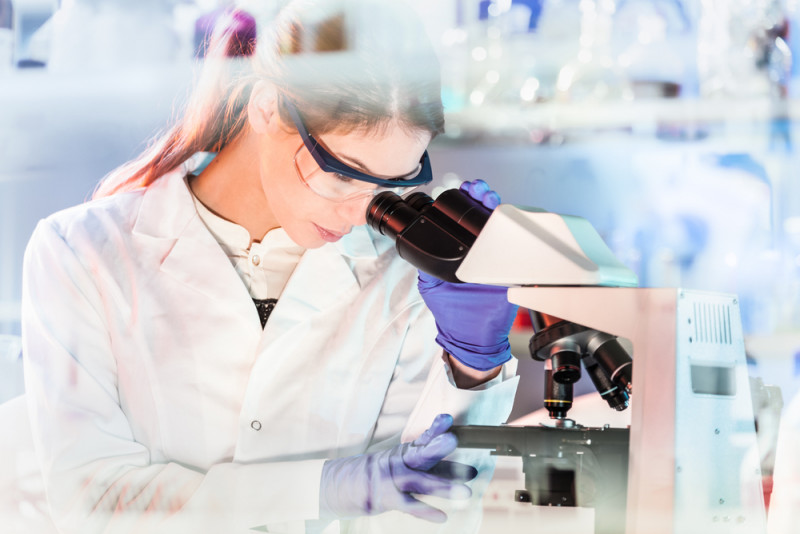
Matej Kastelic/Shutterstock
A new and easy-to-use algorithm may help physicians diagnose epidermolysis bullosa aquisita (EBA) sooner, a process that now can take months or even years.
In a recent study, researchers used artificial intelligence to analyze entire skin biopsies, of which the human eye normally can observe only a small part.
The study, “Use of convolutional neural networks for the detection of u-serrated patterns in direct immunofluorescence images to facilitate the diagnosis of epidermolysis bullosa acquisita,” was published in the American Journal of Pathology.
EBA is a rare subtype of epidermolysis bullosa, in which the body develops antibodies against the essential skin protein type VII collagen.
The antibodies collect in the basement membrane zone, a structure sandwiched between the top two skin laters — epidermis and dermis. They produce a serrated U-shaped pattern that is a key diagnostic feature of EBA.
“However, you need to find and recognize this pattern in a relatively large microscopic slide,” Joost Meijer, MD, PhD, one of the study’s lead investigators, said in a press release.
This pattern occupies a relatively small portion of the typical biopsy, making it hard to find by eye, even under a powerful microscope. It may take up only a 30 x 30 pixel space, for instance, in a digitized microscopic image of 20,000 x 12,000 pixels.
Meijer and his colleague, Astone Shi, PhD, both at the University of Groningen, in the Netherlands, are the lead authors of a study describing an artificial intelligence system capable of analyzing an entire biopsy. It uses convolutional neural networks (CNN), a state-of-the-art medical image analysis technology used in various medical specialties.
“One challenge was that there is no standard training program for this particular pattern,” Shi said.
The researchers adapted and trained nine CNNs to recognize the EBA-specific patterns using 42 skin biopsies. They then validated their procedure using another four patient biopsies. From the total 46 biopsies, 23 were from EBA patients and the others from individuals with other autoimmune skin-blistering disorders.
The best-performing CNN had a 89.3% ability in correctly differentiating EBA cases, outperforming rates published by a group of pathologists and dermatologists, and nearly reaching the accuracy of a small number of highly trained specialists.
“This means that our system outperforms most doctors,” Shi said, likely because the automated process analyzes all tissue fixed to a microscope slide, whereas a human observer takes into account only a relatively small portion of that slide.
“The duration of pattern recognition and classification has not been assessed before, but it can be argued that the automatic tool will always be faster,” the team wrote.
“The proposed approach can assist clinicians and pathologists in recognition of u-serrated patterns in [microscopic] images,” the investigators added, “and facilitate diagnosis of EBA.”
Although the new system is easy to use and performed well in this study, the scientists noted their findings need to be confirmed in a larger dataset.
A prospective European study has launched to that end.
“It will take a year to collect the data of new skin biopsies,” said Meijer, “which will be able to validate the system. Hopefully, we will then have a quicker and easier way to diagnose EBA and prevent the sometimes debilitating scarring.”